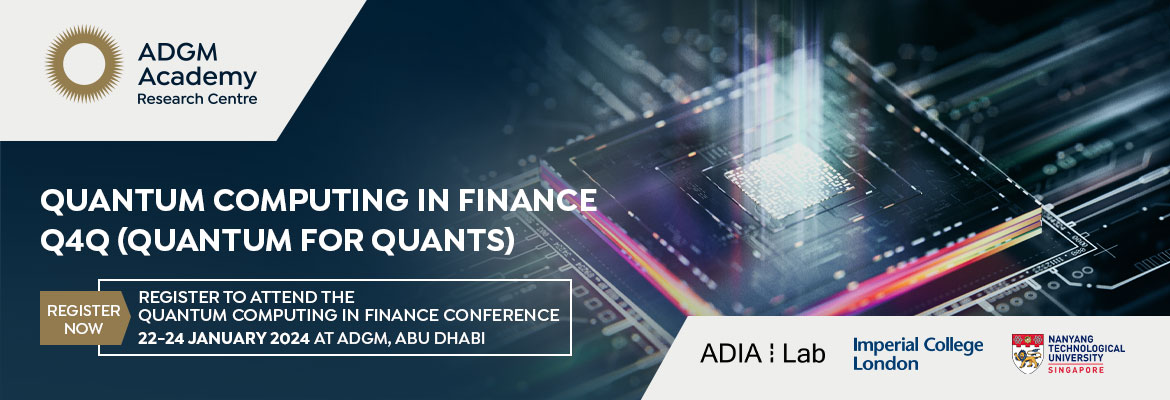
Event Agenda: 23 January 2023 – Day 2
Time
Agenda
08:30 - 09:00
Registration
09:00 - 09:05
Welcome address
Speaker
Horst Simon, ADIA Lab
09:05 - 09:50
Advances in trapped ion qubit technology
Speaker
Christopher Monroe, Duke University (TBC)
09:50 - 10:30
Variational quantum optimization solver
We present a variational quantum optimization solver with polynomially fewer qubits than in standard encodings. We deploy it in IonQ large optimization instances (up to 2000 binary variables) and solve experimentally up to high approximation ratios (significantly above the SDP-relation limits). We believe it is the first experiment with such large instances and such high approximation ratios.
Speaker
Leandro Aolita, Technology Innovation Institute
10:30 - 11:00
Coffee Break
11:00 - 11:30
Quantum feature maps - aspects of theory and hardware implementation
- Quantum kernels and quantum feature maps
- Analogy to other classical models
- The ansatz on NISQ devices
- A simple applied example
Speaker
Marco Paini, Rigetti Computing
11:30 - 12:00
Time-series forecasting with quantum-enhanced signature kernels
- Predicting time series is an ubiquitous task in quantitative finance and, in this talk, we examine a problem defined on real-world financial time-series
- We illustrate a technical approach to time-series predictions based on classical signature kernels combined with quantum feature maps
- We present the results we obtained running the method on Rigetti's quantum computing platform and comparing against classical benchmarks
- We complete the discussion with conclusions and considerations on future potential enhancements and extensions
Speaker
Mattia Fiorentini, Rigetti Computing
12:00 - 12:30
Saving resources with Quantum Agents
Agents often execute complex strategies - continually adapting their reactions to input stimuli to synergize with past actions. As society pushes to automate ever more complex tasks, the computational resource requirements of such agents are growing in tandem – contributing to chip shortages, and a growing energy footprint of such technologies. Indeed, with the rapid advances in large language models, the memory resources costs required by such technologies has been doubling every 3-4 months and energetic costs are growing in tandem. In this talk we determine the fundamental limits on the amount of memory and energy required for executing a complex strategy classically. We demonstrate that a quantum agent can use less memory and lower energetic cost to execute such tasks, implying that it is more efficient to make decisions quantum mechanically.
Speaker
Jayne Thompson, Institute of High Performance Computing
12:30 - 13:30
Lunch
13:30 - 14:00
Quantum computing for probability distributions classification
- Definition of the problem of probability distributions classification and its relevance in finance
- Classical methods and definition of the quantum computing approach to the problem
- Executions on Rigetti's quantum computing platform and results
- Conclusions and outlook
Speaker
David Garvin, Rigetti Computing
14:00 - 14:30
Quantum computing for the pricing of classical and quantum assets
Quantum computers have the potential to provide advantages for solving problems in finance. The talk first reviews the pricing of financial derivatives using quantum subroutines for Monte Carlo estimation. Next, the talk considers a linear algebra scenario of the pricing problem, which naturally includes the setting of incomplete markets. Here, it is shown that a variety of quantum algorithms can be applied, including for quantum algorithms for solving of zero-sum games and the quantum linear systems solver. Finally, the talk defines the notion of quantum assets and develops an extended definition of arbitrage. A quantum version of the first fundamental theorem of asset pricing is provided.
Speaker
Patrick Rebentrost, Centre for Quantum Technologies
14:30 - 15:00
Randomized semi-quantum matrix processing
We apply our technique to four specific use cases: partition-function estimation via quantum Markov-chain Monte Carlo and via imaginary-time evolution; end-to-end linear system solvers; and ground-state energy estimation. For these cases, we prove advantages on average depths, including quadratic speed-ups on costly parameters and even the removal of the approximation-error dependence. All in all, our framework provides a pathway towards early fault-tolerant quantum linear algebra applications.
Speaker
Thais de Lima Silva, Technology Innovation Institute
15:00 - 15:30
Coffee Break
15:30 - 16:30
Panel discussion
Speaker
[TBC]